The Fractal Grid: Part III
The tyranny of peak load – Data is the new copper – Telemetry hardware
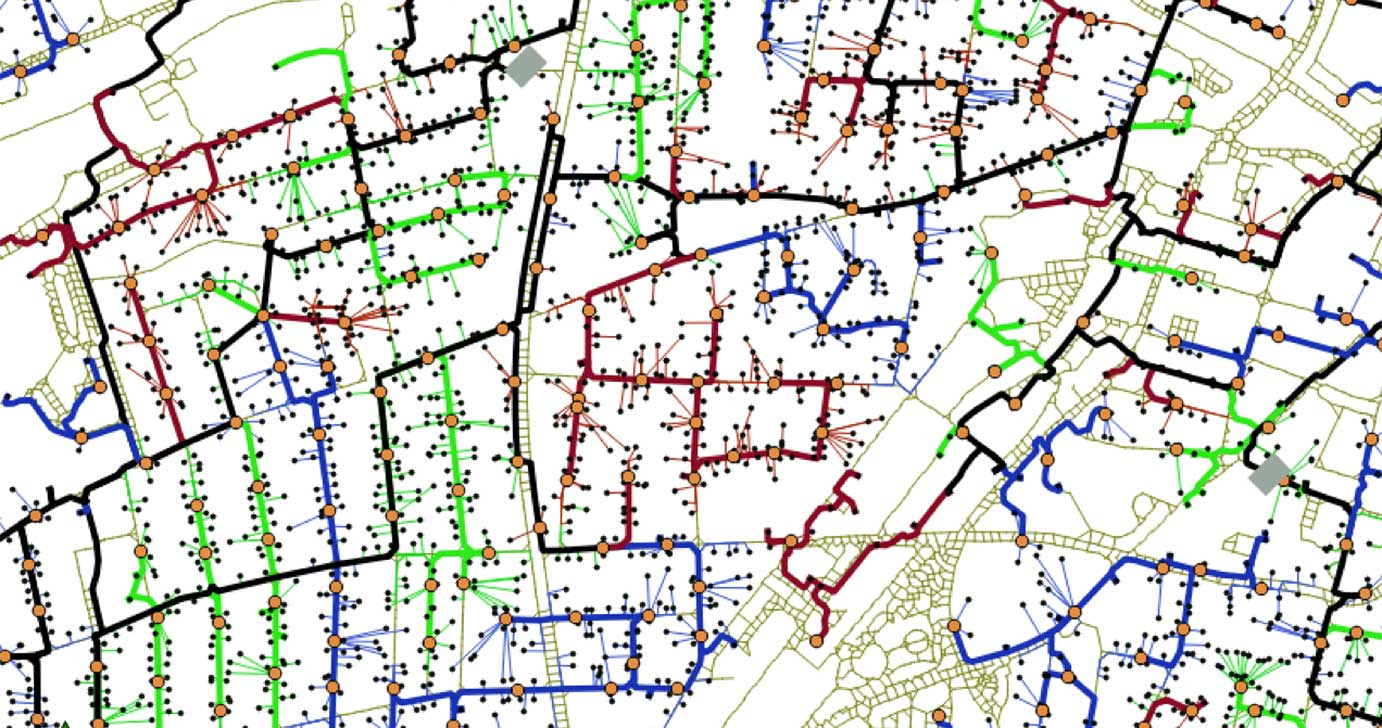
As mentioned in Part II, unlocking the full value that Distributed Energy Resources (DERs) can provide to the distribution system depends on real-time awareness of infrastructure constraints. In turn, the Distribution System Operator’s (DSO) degree of visibility into these constraints and operating parameters depends on how well they can infer the state of the electrical network from available data, a technique called “state estimation”. The quality and comprehensiveness of the data generated from the system’s telemetry equipment is paramount to determining how close the DSO’s digital representation captures the physical reality of the network.
The ultimate goal of state estimation is to establish electrical connectivity between nodes in the network. In other words, the inferred state needs to reconcile the electrical relationships between all the meter sockets, secondary wires, service transformers, primary wires, fuses, jumpers, switches, feeder breakers, substation buses, and finally, substation transformers in the distribution network, each of which has the ability to redirect or modify power flow and therefore change relationships. This level of awareness is critical for system optimization because it enables the DSO to attribute value and fair compensation to load shaping. Without knowing which feeder breaker a home is drawing power from at the moment the price signal is sent, the DSO cannot determine whether load shaping from the home’s battery would alleviate the target constraint or have unintended impacts elsewhere. Without detailed state estimation, DSOs don’t know whether DERs are doing what they are getting paid to do.
Today, utilities have selective real time visibility into important larger junctures in the network, like substations and some medium voltage switches, but minimal real time visibility into meter data at final points of consumption and often no visibility at all into the intermediate nodes in the network, like secondary transformers and line switches. Some utilities have not even rolled out AMI across their full service territories (currently around 75% of consumers have AMI) and even fewer make real-time usage data available to customers (only 2.9% of federally-funded smart meters have this capability, according to Mission: Data).
Topological awareness – monitoring the path and status of lines – is even more desolate, as the geo-coordinates of every line and status of every switch and feeder breaker are often unrecorded or belately recorded in digital models of the network. Some electrical infrastructure generates data from Supervisory Control and Data Acquisition (SCADA) systems and can be updated remotely, but most of the essential and sometimes improvisational adjustments that linemen make to jumpers, switches, and elbows on a week-by-week basis evade the digital model, despite having severe implications for electrical topology and connectivity. These challenges grow with the complexity of the network; the percentage and quality of telemetry coverage deteriorates as the quantity of nodes, interlocking switches, and junctures increase. Some feeders even tie back to themselves. Any digital model hoping to lay the foundations for state estimation must accurately capture this complexity and quickly respond to every lineman, tree, or snowplow that advertently or inadvertently changes topology (and therefore power flow).
However, the biggest challenge could be that the hardware that has been deployed might not be capable of generating data with sufficient granularity to establish electrical connectivity between nodes. Most smart meters and SCADA systems are asynchronous, meaning they can measure volt and amp magnitudes but not the phase angles of the alternating voltage and current. Some argue that asynchronous measurements can only determine how much power is flowing through a given node, not where that electricity is coming from; therefore only synchronous measurements, which record phase angles, can enable the DSO to determine which upstream transformer a meter is electrically connected to at a given point in time.
Phasor Measurement Units (PMUs) are a telemetry technology that records voltage, current angles, and magnitudes of every present electric phase of an alternating current at a frequency measured in milliseconds. Theoretically, assuming there is a PMU at every node, electrical connectivity between nodes can be calculated by comparing their phase angles, making it possible to reliably link meters to upstream feeder breakers or transformers. Although PMUs have actually been around longer than their more prevalent counterparts, they are more expensive, and as a result only a fraction of smart meters and telemetry hardware installed by utilities have the capability to take synchronous measurements.
Due to these data and telemetry challenges, state estimation has largely been confined to academic papers and the transmission system. On the bulk grid, every node above 230 kV is equipped with the telemetry equipment needed to produce input data for the power system optimization software used by ISOs. Although it is unclear what connectivity awareness exists below the microgrid demarcation, resiliency service providers at the consumer level always install the requisite telemetry and controls required to ensure 99.99% uptime at reasonable cost to the socially-essential hospitals and… hydroponic cannabis farms they service. Even residential products sold by companies like Span and Sense market visibility within the home as a defining feature and selling point.
The distribution grid represents the hardest technical and collective action problem. The smaller the lines, the more likely switches are opened and closed without any digital representation and the less likely telemetry coverage is close to sufficient. As a result, distribution planning runs primarily via static reports, DER interconnection is done via manual, bespoke projects, and real time operation is highly conservative and ruled by the extremes at the upper tail of the distribution. With minimal awareness, operators responsible for reliability have to assume the worst, creating tyranny by static, maximal ratings where constraints and their corresponding price signals are updated infrequently and set almost exclusively by peak load events.
However, some startups are betting that advances in software and machine learning can help distribution system operators roll back a bit of fogginess and capture unrealized value even without PMUs at every node.
Kevala provides data-driven distribution planning services for utilities, and in doing so, is amassing the data required to build a "1:1" map of the grid capable of running power flow analysis down to the service point level, a critical first step towards state estimation. It seems natural that automated interconnection and active network management would live within the same data ecosystem, and as outlined in “The Digital Rate Case” by Jake Jurewicz, analyzing, siting, approving, and efficiently managing DERs via one service offering is a great product experience for developers, utilities, and regulators alike.
Camus, like Kevala, is selling directly to infrastructure owners but focused on real-time management for smaller municipally-owned utilities before expanding to investor-owned behemoths. Inspired by recent advances in distributed computing, their product is focused on providing the operational visibility and decentralized control required to coordinate DERs owned by multiple independent parties.
Both companies, as well as established players like Palantir and GoogleX, are approaching the visibility gaps from different angles and could eventually be well-positioned to approximate the state of a distribution network with enough granularity to realize the vision of a fractal distribution grid, especially with potential hardware partners like SunRun and Sunnova already planning on building community microgrids. However, state estimation will advance the frontier of awareness only to the point where the marginal cost of additional granularity exceeds the marginal value provided by intelligent DER coordination. Some see distribution-level price signals as contingent on complete deployment of PMU telemetry because without the ability to couple meters to upline feeder breakers and transformers, the DSO cannot have faith that a specific action will alleviate the targeted constraint nor could they properly compensate DERs for load shaping behavior. Regardless, the point of diminishing returns may arrive sooner than anticipated unless utilities install more telemetry hardware. Even then, the overhead required to continuously run these types of markets at the distribution level might be cost-prohibitive.
Despite the technical and economic risks, the biggest open question of all rests with institutional barriers to entry. Ultimately, we can never know if this vision will work without the buy-in from those who own, operate, and control distribution infrastructure in the US: utilities. Do they have the capability, and the motivation, to build the distribution grid we need?
Continued in Part IV.